The content of this page has not been vetted since shifting away from MediaWiki. If you’d like to help, check out the how to help guide!
Introduction & Overview
The BigStitcher is a software package that allows simple and efficient alignment of multi-tile and multi-angle image datasets, for example acquired by lightsheet, widefield or confocal microscopes. The software supports images of almost arbitrary size ranging from very small images up to volumes in the range of many terabytes, which are for example produced when acquiring cleared tissue samples with lightsheet microscopy.
As its predecessor, the Image Stitching, the BigStitcher can run in fully automatically mode but is additionally able to guide the user through the alignment process by interactively showing intermediate results with the option for user interaction, which includes interactive setup of approximate tile locations. The BigStitcher is fully integrated with the BigDataViewer and is thus able to interactively display and process the input images independent of their size. An improved global optimization is now able to efficiently align sparsely connected datasets, where image content is separated by large areas of almost constant background.
In addition to the documentation here there is a growing set of tutorial videos on this YouTube Channel
Specifically intended for lightsheet acquisitions of cleared samples (e.g. by CLARITY), the BigStitcher offers several features:
- Registration of multi-view multi-tile acquisitions, where each view of the sample that is acquired from different orientations may consist of many individual image tiles.
- Integrated downstream processing of the data such fusion and deconvolution of the aligned data at different resolution levels (full or reduced resolutions) and for selected areas (full image or selected parts)
- Since cleared samples are typically acquired by a lightsheet microscope that can illuminate from different directions, the BigStitcher supports a pre-selection of the best illumination direction at every image block in the sample
- Support of non-regular acquisition grids, which includes ‘intelligent acquisitions’ where some of the image blocks can be missing if only background is present
- Support of image data acquired at different resolutions to combine overview scans with high resolution acquisitions of specific areas of interest
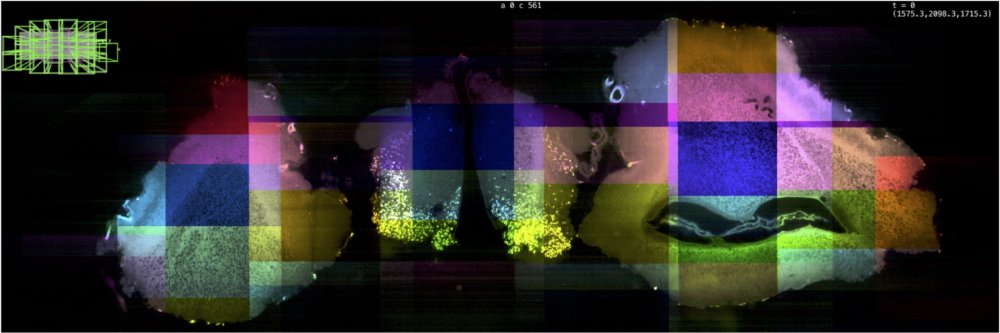
Download
A beta-Version of BigStitcher is available via the Fiji Updater. Just go to Help › Update…, click Manage update sites
and select BigStitcher
in the list. After applying the changes and restarting Fiji, BigStitcher will be available under Plugins › BigStitcher › BigStitcher.
The source code is available on GitHub, please also report feature requests & bugs there.
Documentation
The workflow of the BigStitcher consists of multiple steps covering import, visualisation, processing, and output that are explained in full detail under the respective pages linked below:
Opening / Importing an Image Dataset
Since image data is produced in a variety of formats, the first step is to assemble all images into a dataset and load the necessary metadata or manually define it if it is missing. The BigStitcher is integrated with the BigDataViewer and thus shares the same data representation, which is stored as a XML file on disk.
Once a dataset was defined and the XML file was saved, you can quickly load any dataset:
UI Overview
The BigStitcher can run in two modes depending on whether you want to align tiled images imaged from the same direction (Stitching mode) or align tiled acquisitions from different angles or time points (MultiView mode). The following sections give an overview of the UI in both modes:
Pre-alignment
Ideally, the location of tiles should have been loaded from metadata while importing a dataset. With the BigStitcher you can, however, also move views to a regular grid manually, load view locations from a tile configuration file or manually translate single views.
Many lightsheet microscopes offer illumination of the sample from multiple directions. Since typically one illumination direction produces the best image for a given point in the sample, you might want to discard images illuminated from other directions.
Furthermore, you might want to correct your images for uneven brightness across the field-of-view or camera offsets (“flatfield correction”). We offer on-the-fly flatfield correction as an experimental feature.
Quality Control
To quantify image quality throughout a dataset, we allow for the calculation of (relative) Fourier Ring Correlation (FRC) in the images. You can use this functionality to assess whether image quality/resolution is constant throughout the sample or it decreases, e.g. as you go deeper into the sample.
Stitching
Stitching consists of aligning multiple overlapping image tiles using a translation model. If your dataset consists of tiled acquisitions from multiple angles, the stitching should be done for all angles independently before aligning the angles using Multiview Reconstruction:
The basic stitching pipeline consists of 3 steps:
Additionally, we offer the possibility to refine the alignment with a more complex affine transformation model using the iterative Closest Point (ICP) algorithm:
In addition to the standard stitching pipeline, we also offer advanced functionality, such as different algorithms for determining pairwise shifts:
MultiView registration
Once you have aligned the views imaged from the same angles to each other, you can then proceed to align those groups of views to each other, using Multiview-Reconstruction. For this, switch to MultiView mode in the main window.
This process consists of finding interest points such as nuclei or fiducial beads in the single views, matching corresponding interest points in other views and then aligning the views based on the corresponding interest points.
In MultiView mode, we also offer various ways of visualizing and managing interest points:
Blocked and Non-Rigid Refinement
In some cases, especially when aligning images illuminated from different sides or rotated views, even affine registration with MultiView registration or ICP might still produce suboptimal results. We support two ways of increasing registration quality further.
The first improvement is to split the images of the dataset into virtual blocks, allowing for a more fine-grained registration using the same registration tools available for the whole images:
Fusion
Once the views in your dataset have been aligned, a typical last step is to create a “classical image” that can be used by other ImageJ-plugins or other programs altogether. The process of merging multiple aligned images into one resulting image is called Image Fusion:
Often, you might not want to fuse the whole sample, but rather a smaller sub-volume (defined by a Bounding Box). To do this, you have to define a bounding box to use in the fusion:
All bounding boxes will be axis-aligned in the global coordinate system of your dataset (determined by the coordinate system of the first view). Sometimes, the sample is “tilted” in respect to this coordinate system, leading to unnecessarily big dark space around the sample in the fused image. We therefore offer various ways of re-orienting the sample for more space-efficient fusion:
If the brightness of the various Views/Tiles in you dataset differs (e.g. due to bleaching), you have the option of determining an optimized brightness and contrast adjustment that will be applied during the fusion process:
(MultiView) Deconvolution
In addition to simple view fusion, we can additionally perform Deconvolution of the resulting images, ideally using a Point Spread Function (PSF) extracted from the input images themselves (e.g. if they contain sub-diffraction-sized fluorescent beads).
The first step in this process is assigning PSFs to the views you want to deconvolve:
After assigning PSFs to all the views you want to process, you can proceed with the deconvolution itself:
Headless Operation
We provide a macro-scriptable version of most processing steps in BigStitcher. They can be recorded using the Macro Recorder and the resulting script can be adapted, e.g. for batch processing.
Example Datasets
We prepared a 2D and 3D version of a tiled dataset for testing the BigStitcher on a small example. We suggest to run BigStitcher on these first before applying it to your dataset. This allows you to quickly test features in an environment where you can easily ask for advice on GitHub or the ImageJ Forum. We will add a larger, multi-view, dataset as a showcase item in the future.
2D multi-tile dataset (2.8 MB)
This dataset is a maximum intensity projection of the nervous system of a Drosophila larva containing 6 tiles and 3 channels each. You can download the raw input at http://preibischlab.mdc-berlin.de/BigStitcher/Grid_2d.zip> and a reconstructed BigStitcher project at <http://preibischlab.mdc-berlin.de/BigStitcher/Grid_2d_h5_aligned.zip. In the reconstructed project, the images were imported into the BigStitcher using the AutoLoader (with immediate resaving as HDF5 and Movement to a regular 2-by-3 grid with 10% overlap between the tiles). We calculated pairwise shifts using phase correlation with default parameters, using the precomputed 2x2 downsampling and averaging the channels. We ignored links with correlation \(<0.7\) and calculated the final registration using the two-round global optimization with strict constraints.
3D multi-tile dataset (123 MB)
This dataset is a 3d confocal scan of the nervous system of a Drosophila larva containing 6 tiles and 3 channels each, channels are distributed over different files. You can download the raw input at http://preibischlab.mdc-berlin.de/BigStitcher/Grid_3d.zip> and the reconstructed project at <http://preibischlab.mdc-berlin.de/BigStitcher/Grid_3d_h5_aligned.zip. In the reconstructed project, we ran the same import and reconstruction steps as for the 2d dataset and in addition performed affine refinement of the registration using IPC with default parameters and simple tile refinement to create the final reconstructed project.